The Use of Artificial Intelligence at MLL Munich Leukemia Laboratory
Whether a search engine or digital assistant is involved, artificial intelligence (AI) has long been a part of our day-to-day work. There are success stories in imaging-based diagnostics thanks to AI as well. Preliminary studies, for example, indicate that the classification of medical findings using AI is equivalent to traditional methods*. While AI will never replace medical work, it will support it in significant ways. What this can look like in specific cases and how MLL utilizes the potential of AI to advance hematological diagnostics can be seen from application examples from the MLL departments.
To begin with, AI must learn to identify characteristic features in a dataset. In a training phase, the AI runs through iterative cycles of training and validation until it is finally able to recognize the relevant characteristics autonomously and precisely and to produce a classification. It is also true of AI that practice makes perfect – the training dataset just needs to be big enough.
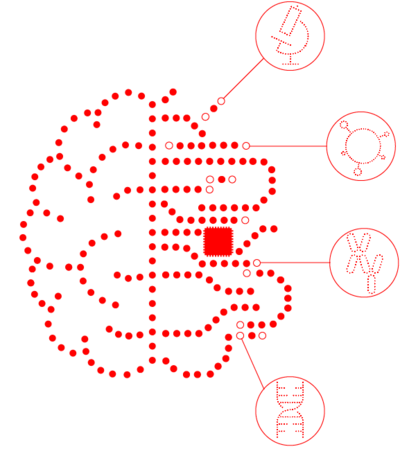
Cytomorphology
In the area of cytomorphology, MLL is working to prepare a database that will initially include around half a million annotated single-cell images of peripheral blood cells. Using this, AI will learn to prepare a differential blood count based on blood smears in preparation for the MTA. Planned as the next step is the automated analysis of bone marrow.
Chromosomal Analysis
AI has already been supporting MLL in this since the summer of 2019. Based on metaphase images, AI identifies chromosomes, isolates them, and places them in the correct order and orientation in the karyogram. Each karyogram prepared in this way is carefully checked, and any errors are rectified manually. AI support saves a lot of time, which the employees can use for interpreting the data and characterizing complex aberrations. Together with its cooperation partner MetaSystems, MLL is working on continuously improving AI for conventional chromosome analysis.
Immunophenotyping
AI used for this application, was developed jointly with the working group of Professor Krawitz (University of Bonn) and is already proving to be a specialist in classifying mature B-cell neoplasias. In a feasibility study conducted over several months, the analysis of raw data from routine flow cytometry assays was compared in parallel and independently by the MTA, physicians/biologists as well as by AI. So far, AI only makes predictions concerning the diagnosis instead of producing findings, but it also indicates how probable the specific diagnosis is and which one would be the second most probable. At a predictive confidence of >95%, AI correctly detected the presence of mature B-cell neoplasia in 99.7% of cases and also provided a correct class allocation 99.3% of the time (Kern et al. 2019 ASH Abstract 886). At present, the goal is to expand the diagnostic spectrum to all other areas of immunophenotyping, including marrow/cortex differentiation using AI.
Molecular Genetics
Evidence of mutations in a gene panel of interest can be of great importance for diagnosis, risk assessment and even for the selection of therapies for hematological neoplasias. However, the interpretation of detected gene variants poses a considerable challenge in many cases – because not every gene variant is relevant to disease. The AI tool developed at MLL, called the “MLL Predictor”, helps physicians and scientists to assess whether a gene variant is pathogenic or benign or if its significance is still unclear based on the currently available worldwide databases (VUS, variant of unknown significance).
In addition, studies show that not only individual mutations but also patterns of mutations can be characteristic of certain diseases. For this reason, especially against the backdrop of genome-wide data, AI will very soon be irreplaceable when it comes to identifying these patterns of mutations. AI-based classification models that include these patterns are already providing a great deal of assistance with differential diagnostics (Meggendorfer et al. 2019 ASH Abstract 4663, Baer et al. 2019 ASH Abstract 2735).
Further references
*Hu et al. JCNI 2019, Scheib et al. Radiology 2019, McKinney et al. Nature 2020